Modernisation is key to enabling sustainable and economic growth in intelligent automation
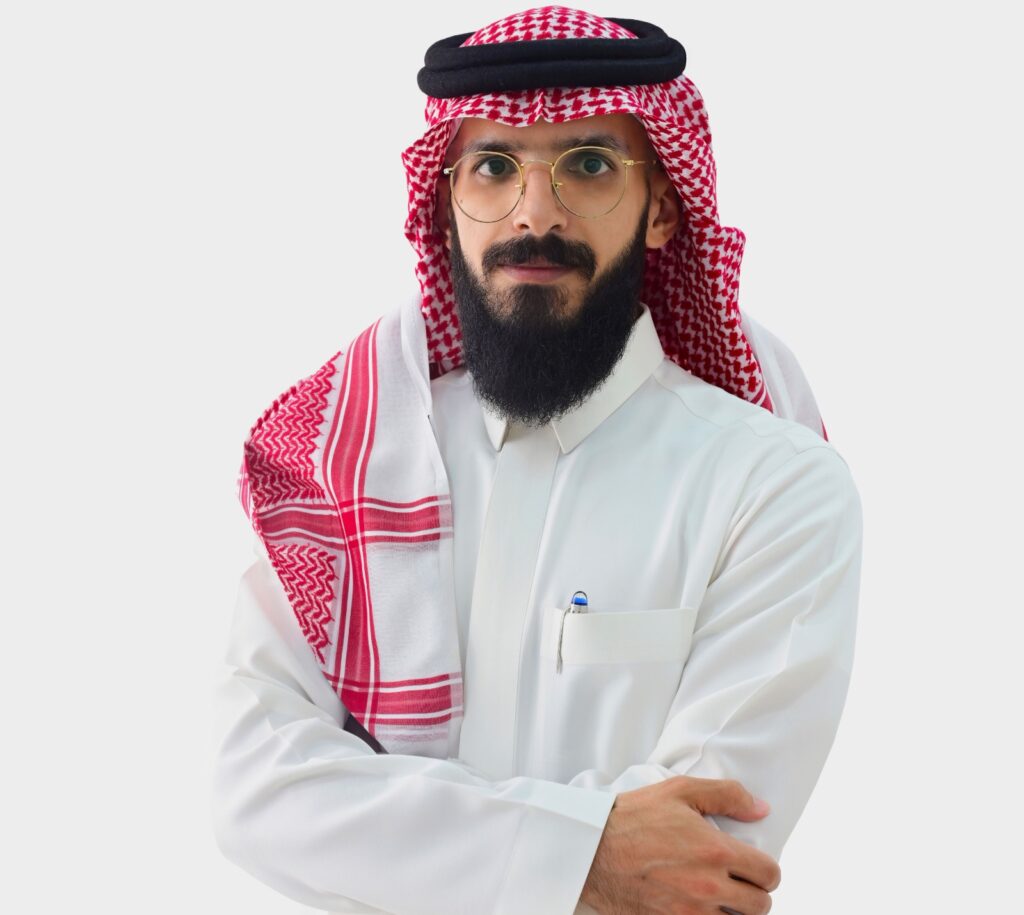
Why AI is Piling Pressure on Creaking IT Infrastructures
Modernisation is key to enabling sustainable and economic growth in intelligent automation
Author: Hamad Alkathiri,
Senior Systems Engineer, Public Sector – Saudi Arabia at Nutanix
It’s been barely a year since the launch of ChatGPT and yet generative AI is now on the lips of most business execs. GenAI has been what Forrester CEO George Colony recently referred to as “the most important technology thunderstorm of the last 40 years,” and tech leaders across the Europe, Middle East and Africa (EMEA) region seem to agree. AI is now a priority for 90% of IT, DevOps, and platform engineering decision makers, at least according to the Vanson Bourne and Nutanix State of Enterprise AI Report [link]. But as with any rapid advance in technology, questions start to be asked of the existing IT infrastructure and its ability to cope. Are current systems really up to the demands of an AI gold rush?
The short answer is no.
To begin with, we have an energy efficiency problem. This is something Gartner warned about back in January, saying that by 2025 “AI will consume more energy than the human workforce, significantly offsetting carbon-zero gains.” Also a recent study called the Growing energy footprint of AI, suggested that the AI industry could consume as much energy as a country the size of the Netherlands by 2027.
The need to address, or at least the need for ESG reporting considerations to be addressed, was highlighted in the State of Enterprise AI Report. Today most AI/ML model inferencing and training is conducted on high-performance GPUs, which are supported by equally high-performance memory and storage. Combined, these solutions consume significant amounts of electricity and require additional power to actively cool within a private or public data centre.
The report also identified additional challenges around skills. Gaps in AI capabilities, as well as ESG, are big concerns. Over 40% of EMEA respondents in the report claim they lack GenAI and prompt engineering skills, and desperately need data scientists. This will inevitably impact organisations’ ability to meet their own expectations with AI projects.
This becomes even more pronounced when considering other major factors such as managing data and scalability in back-office functionality. Skills shortages and a need to modernise systems do not go well together, so addressing skills is important in meeting the on-going demands of infrastructure change.
This is the modern world
AI will only add pressure to existing systems, so there is also growing recognition of the need to address the managing and support of running AI workloads at scale. In fact, EMEA respondents ranked this as the number one challenge over the next two years. In addition, respondents also cited security, reliability and disaster recovery as important considerations in their AI strategy.
With infrastructure modernisation and data security outranking cost (the third-lowest consideration for EMEA organisations running or planning to run AI workloads), there is a clear indication within the region that organisations recognise that to really benefit from AI they have to get their infrastructure house in order.
This is further illustrated by the report, with over 90% of EMEA respondents agreeing that their IT costs and cloud spending will both increase due to AI applications. In short, EMEA organisations are showing a willingness to spend in support of their AI initiatives. The challenge is where and how to spend it wisely.
It will come down to prioritisation. While identifying and remediating skills shortages are a constant issue, especially when it comes to emerging technologies, infrastructure modernisation is key. AI applications and services have a symbiotic relationship with their underlying datasets, models, and infrastructure. This is something the report shows that enterprises are acutely aware of, so the challenge is how to develop data security and quality strategies to make their AI technology as reliable and resilient as possible.
Inevitably, the gold rush nature of GenAI adoption will lead to some short-term over-spend to plug skills gaps and deliver infrastructure capabilities. However, a longer-term modernisation plan is needed to really benefit from the technology, to ensure scalability and intelligent workloads that optimise costs and energy use. This will mean effective implementation and management of data across multiple environments – data centre, cloud, and edge – as each will play a critical role in supporting an end-to-end AI workflow.
This data management should also consider security, data quality and data protection. Given data sovereignty requirements, especially in the EMEA region, this should be a core tenet of any AI strategy. Of course, this is all a work in progress. Organisations are still trying to work out how best to use GenAI but use it they will. Inevitably, there will be early adopters, accelerating adoption and making mistakes along the way but for the majority there are some fundamentals here. Existing infrastructures are not enough. They will creak and fail under the strain of AI, if not physically then almost certainly in terms of capability and governance. Thankfully, on that score, for most organisations, AI will be a marathon, not a sprint.