AI and ML reliability and security: BlenderBot and other cases
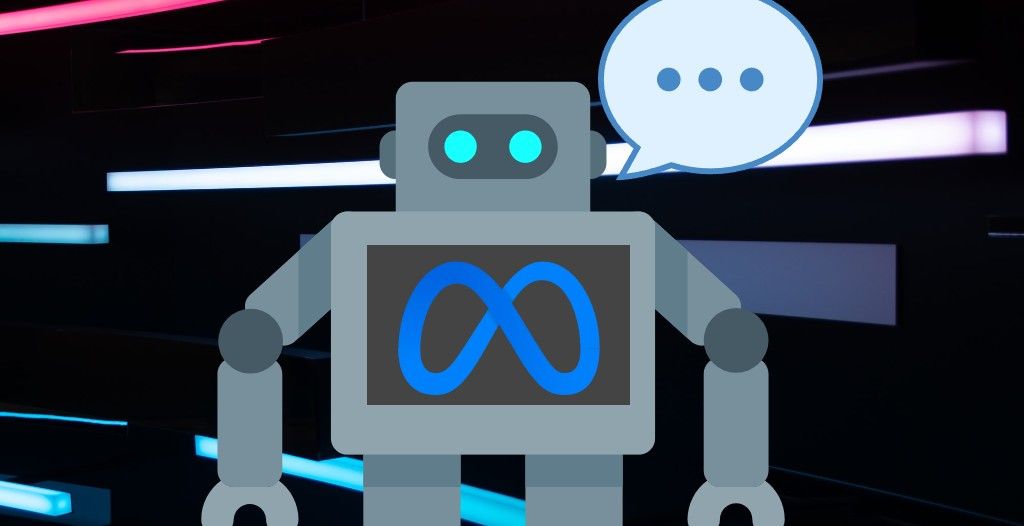
Since its launch in early August 2022, Blenderbot, an AI-driven research project by Meta, has been hitting the headlines. Blenderbot is a conversational bot, and its statements about people, companies or politics appear to be unexpected and sometimes radical. This is one of the challenges with machine learning, and it is important that organizations using ML in their business deal with it.
Other similar projects previously faced the same problem that Meta did with Blenderbot, such as, Microsoft’s chatbot Tay for Twitter, which ended up making racist statements. This reflects the specifics of generative machine learning models trained on texts and images from the internet. To make their outputs convincing, they use huge sets of raw data, but it is hard to stop such models from picking up biases if they are trained on the web.
By now, these projects have mostly research and science goals. However, organizations also use language models in practical areas, such as customer support, translation, writing marketing copy, text proofreading and so on. To make these models less biased, developers can curate the datasets used for training. However, this is very difficult in the case of web-scale datasets. To prevent embarrassing errors, one approach is to filter data for biases, for example, using particular words or phrases to remove the respective documents and prevent the model from learning on them. Another approach is to filter out inappropriate outputs in case model generates questionable text before it reaches users.
Looking more broadly, protection mechanisms are necessary for any ML model, and not only from biases. If developers use open data to train the model, attackers can exploit this with a technique called “data poisoning,” where attackers add specially crafted malformed data to the dataset. As a result, the model will not be able to identify some events or will mistake them for others and make the wrong decisions.
“Although in reality such threats remain rare as they require a lot of effort and expertise from attackers, companies still need to follow protective practices. This will also help minimize errors in the process of training models,” comments Vladislav Tushkanov, Lead Data Scientist at Kaspersky. “Firstly, organizations need to know what data is being used for training and where it comes from. Secondly, the use of diverse data makes poisoning more difficult. Finally, it is important to thoroughly test the model before rolling it out into combat mode and constantly monitor its performance.”
Organizations can also refer to MITRE ATLAS – a dedicated knowledgebase to navigate businesses and experts through threats for machine learning systems. ATLAS also provides a matrix of tactics and techniques used in attacks on ML.
At Kaspersky, we conducted specific tests on our anti-spam and malware detection systems by imitating cyberattacks to reveal potential vulnerabilities, understand the possible damage and how to mitigate the risk of such attack.
Machine learning is widely used in Kaspersky products and services for threat detection, alert analysis in Kaspersky SOC or anomaly detection in production process protection. To learn more about machine learning in Kaspersky products, visit this page.