AI Won’t Save You Unless Your Business Architecture Is Ready
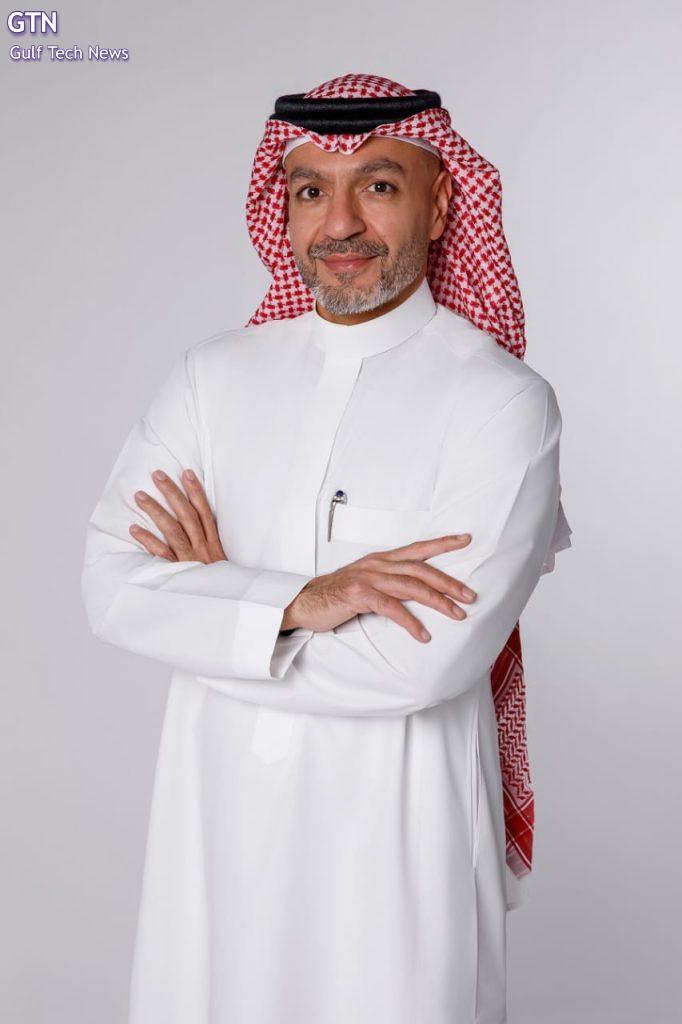
For Gulf Tech News
By Mamdouh Allam, General Manager for Splunk
Artificial intelligence is, quite appropriately, being embraced across industries as a transformative force. From national strategies, to enterprise investments in smart infrastructure and sovereign data platforms, there is no shortage of ambition. Yet I can’t help but feel that many organisations might be missing a critical aspect of their planning when it comes to deploying AI-enabled tools..tools..
Too often, the conversation around AI readiness starts with the tools themselves – acting as though AI can be an answer in-and-of-itself: a self-contained solution, which in some cases it may be. But while the latest generative models and machine learning techniques may be impressive, they are not necessarily a silver bullet. Without the right business architecture and foundation in place, businesses risk hampering the value their AI-enabled systems can deliver.
Many organizations fall into the trap of treating AI as a plug-and-play solution. They pilot a tool, integrate a model, and hire a few data scientists. But they overlook the most critical element: how AI fits into the way the business actually works. Business architecture is the connective tissue between strategy and execution. It defines how people, processes, data, and systems interact to deliver outcomes. If that architecture is fragmented, outdated, or opaque, AI initiatives will struggle. Models will not align with business priorities. Data will be siloed or untrusted. Outputs will be difficult to interpret or act on.
In the race to adopt AI, this is where many organizations stumble. In reality, AI is exposing flaws in business architecture that have long gone unnoticed. It is not the technology that is failing. It is that the business is not ready to support it.
Another barrier to adoption is not regulation, risk, or cost. It is internal doubt. In conversations with customers across sectors, a recurring theme is hesitation. Leaders want to leverage AI, but they are unsure where to begin, how to scale, or whether their data is reliable enough to train a model. This doubt slows innovation. But the path forward begins not with AI, but with data.
Organizations that adopt a “database approach” to AI are the ones making real progress. This means creating a unified, real-time view of data across the organization, supported by governance, observability, and performance monitoring. It means treating data not as a by-product of operations but as the foundation of intelligence. With this foundation in place, confidence grows. AI becomes less of a black box and more of a strategic tool. Security teams can trust anomaly detection models. IT leaders can act on predictive analytics quickly. Executives can make decisions that are data-backed and timely. When organizations trust their data, they trust their AI.
There are countless AI tools on the market, and that abundance creates a new challenge. Faced with too many options, each offering different benefits and use cases, businesses can become overwhelmed. The solution is not to chase every new tool, but to simplify. Simplification does not mean scaling back ambition. It means making sure every AI initiative is tied to a clear business goal, supported by accessible data, and designed to enhance human decision-making.
At Splunk, we see this in action every day. Customers who implement AI successfully in their security or observability operations are not doing it because it is trendy. They are solving real problems. They use AI to reduce time to detect threats, streamline incident response, and identify anomalies before they disrupt operations. These are measurable, repeatable efforts aligned with business priorities.
The global momentum around AI is real, and the vision is bold. But the true test of success will not be how many models are launched or how much infrastructure is built. It will be how deeply AI is embedded in decision-making, operations, and customer experience.
This requires a shift in mindset. AI readiness is not just about having the latest technology. It is about standing back and asking yourself what you really need from your AI, and what the right tools are, developing the right business architecture, assessing your data and fostering a culture of trust in this data, and focusing on outcomes. When organizations take this approach, AI becomes more than just another innovation. It becomes a source of competitive advantage, a growth accelerator, and a contributor to long-term transformation.